Exploring The Evolving Role Of Data Engineers
Data Engineering Podcast - Un pódcast de Tobias Macey - Domingos
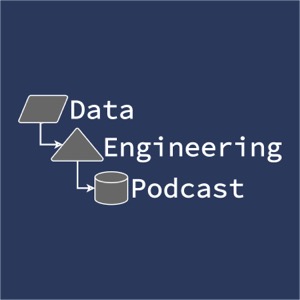
Categorías:
Summary Data Engineering is still a relatively new field that is going through a continued evolution as new technologies are introduced and new requirements are understood. In this episode Maxime Beauchemin returns to revisit what it means to be a data engineer and how the role has changed over the past 5 years. Announcements Hello and welcome to the Data Engineering Podcast, the show about modern data management When you’re ready to build your next pipeline, or want to test out the projects you hear about on the show, you’ll need somewhere to deploy it, so check out our friends at Linode. With their managed Kubernetes platform it’s now even easier to deploy and scale your workflows, or try out the latest Helm charts from tools like Pulsar and Pachyderm. With simple pricing, fast networking, object storage, and worldwide data centers, you’ve got everything you need to run a bulletproof data platform. Go to dataengineeringpodcast.com/linode today and get a $100 credit to try out a Kubernetes cluster of your own. And don’t forget to thank them for their continued support of this show! Struggling with broken pipelines? Stale dashboards? Missing data? If this resonates with you, you’re not alone. Data engineers struggling with unreliable data need look no further than Monte Carlo, the world’s first end-to-end, fully automated Data Observability Platform! In the same way that application performance monitoring ensures reliable software and keeps application downtime at bay, Monte Carlo solves the costly problem of broken data pipelines. Monte Carlo monitors and alerts for data issues across your data warehouses, data lakes, ETL, and business intelligence, reducing time to detection and resolution from weeks or days to just minutes. Start trusting your data with Monte Carlo today! Visit dataengineeringpodcast.com/montecarlo to learn more. The first 10 people to request a personalized product tour will receive an exclusive Monte Carlo Swag box. Are you bored with writing scripts to move data into SaaS tools like Salesforce, Marketo, or Facebook Ads? Hightouch is the easiest way to sync data into the platforms that your business teams rely on. The data you’re looking for is already in your data warehouse and BI tools. Connect your warehouse to Hightouch, paste a SQL query, and use their visual mapper to specify how data should appear in your SaaS systems. No more scripts, just SQL. Supercharge your business teams with customer data using Hightouch for Reverse ETL today. Get started for free at dataengineeringpodcast.com/hightouch. Your host is Tobias Macey and today I’m interviewing Maxime Beauchemin about the impacts that the evolution of the modern data stack has had on the role and responsibilities of data engineers Interview Introduction How did you get involved in the area of data management? What is your current working definition of a data engineer? How has that definition changed since your article on the "rise of the data engineer" and episode 3 of this show about "defining data engineering"? How has the growing availability of data infrastructure services shifted foundational skills and knowledge that are necessary to be effective? How should a new/aspiring data engineer focus their time and energy to become effective? One of the core themes in this current spate of technologies is "democratization of data". In your post on the downfall of the data engineer you called out the pressure on data engineers to maintain control with so many contributors with varying levels of skill and understanding. How well is the "modern data stack" balancing these concerns? An interesting impact of the growing usage of data is the constrained availability of data engineers. How do you see the effects of the job market on driving evolution of tooling and services? With the explosion of tools and services for working with data, a new problem has evolved of which ones to use for a given organization. What do you see as an effective and efficient process for enumerating and evaluating the available components for building a stack? There is also a lot of conversation around the "modern data stack", as well as the need for companies to build a "data platform". What (if any) difference do you see in the implications of those phrases and the skills required to compile a stack vs build a platform? How do you view the long term viability of templated SQL as a core workflow for transformations? What is the impact of more acessible and widespread machine learning/deep learning on data engineers/data infrastructure? How evenly distributed across industries and geographies are the advances in data infrastructure and engineering practices? What are some of the opportunities that are being missed or squandered during this dramatic shift in the data engineering landscape? What are the most interesting, innovative, or unexpected ways that you have seen the data ecosytem evolve? What are the most interesting, unexpected, or challenging lessons that you have learned while contributing to and participating in the data ecosystem? In episode 3 of this show (almost five years ago) we closed with some predictions for the following years of data engineering, many of which have been proven out. What is your retrospective on those claims, and what are your new predictions for the upcoming years? Contact Info LinkedIn @mistercrunch on Twitter mistercrunch on GitHub Parting Question From your perspective, what is the biggest gap in the tooling or technology for data management today? Links How the Modern Data Stack is Reshaping Data Engineering The Rise of the Data Engineer The Downfall of the Data Engineer Defining Data Engineering – Data Engineering Podcast Airflow Superset Podcast Episode Preset Fivetran Podcast Episode Meltano Podcast Episode Airbyte Podcast Episode Ralph Kimball Bill Inmon Feature Store Prophecy.io Podcast Episode Ab Initio Dremio Podcast Episode Data Mesh Podcast Episode Firebolt Podcast Episode The intro and outro music is from The Hug by The Freak Fandango Orchestra / CC BY-SA Support Data Engineering Podcast