Keeping A Bigeye On The Data Quality Market
Data Engineering Podcast - Un pódcast de Tobias Macey - Domingos
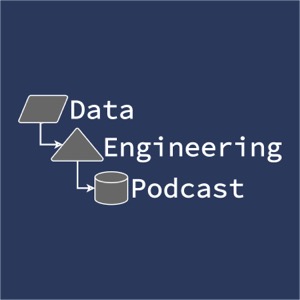
Categorías:
Summary One of the oldest aphorisms about data is "garbage in, garbage out", which is why the current boom in data quality solutions is no surprise. With the growth in projects, platforms, and services that aim to help you establish and maintain control of the health and reliability of your data pipelines it can be overwhelming to stay up to date with how they all compare. In this episode Egor Gryaznov, CTO of Bigeye, joins the show to explore the landscape of data quality companies, the general strategies that they are using, and what problems they solve. He also shares how his own product is designed and the challenges that are involved in building a system to help data engineers manage the complexity of a data platform. If you are wondering how to get better control of your own pipelines and the traps to avoid then this episode is definitely worth a listen. Announcements Hello and welcome to the Data Engineering Podcast, the show about modern data management What are the pieces of advice that you wish you had received early in your career of data engineering? If you hand a book to a new data engineer, what wisdom would you add to it? I’m working with O’Reilly on a project to collect the 97 things that every data engineer should know, and I need your help. Go to dataengineeringpodcast.com/97things to add your voice and share your hard-earned expertise. When you’re ready to build your next pipeline, or want to test out the projects you hear about on the show, you’ll need somewhere to deploy it, so check out our friends at Linode. With their managed Kubernetes platform it’s now even easier to deploy and scale your workflows, or try out the latest Helm charts from tools like Pulsar and Pachyderm. With simple pricing, fast networking, object storage, and worldwide data centers, you’ve got everything you need to run a bulletproof data platform. Go to dataengineeringpodcast.com/linode today and get a $60 credit to try out a Kubernetes cluster of your own. And don’t forget to thank them for their continued support of this show! Modern Data teams are dealing with a lot of complexity in their data pipelines and analytical code. Monitoring data quality, tracing incidents, and testing changes can be daunting and often takes hours to days. Datafold helps Data teams gain visibility and confidence in the quality of their analytical data through data profiling, column-level lineage and intelligent anomaly detection. Datafold also helps automate regression testing of ETL code with its Data Diff feature that instantly shows how a change in ETL or BI code affects the produced data, both on a statistical level and down to individual rows and values. Datafold integrates with all major data warehouses as well as frameworks such as Airflow & dbt and seamlessly plugs into CI workflows. Go to dataengineeringpodcast.com/datafold today to start a 30-day trial of Datafold. Once you sign up and create an alert in Datafold for your company data, they will send you a cool water flask. Are you bogged down by having to manually manage data access controls, repeatedly move and copy data, and create audit reports to prove compliance? How much time could you save if those tasks were automated across your cloud platforms? Immuta is an automated data governance solution that enables safe and easy data analytics in the cloud. Our comprehensive data-level security, auditing and de-identification features eliminate the need for time-consuming manual processes and our focus on data and compliance team collaboration empowers you to deliver quick and valuable data analytics on the most sensitive data to unlock the full potential of your cloud data platforms. Learn how we streamline and accelerate manual processes to help you derive real results from your data at dataengineeringpodcast.com/immuta. Your host is Tobias Macey and today I’m interviewing Egor Gryaznov about the state of the industry for data quality management and what he is building at Bigeye. Interview Introduction How did you get involved in the area of data management? Can you start by sharing your views on what attributes you consider when defining data quality? You use the term "data semantics" – can you elaborate on what that means? What are the driving factors that contribute to the presence or lack of data quality in an organization or data platform? Why do you think now is the right time to focus on data quality as an industry? What are you building at Bigeye and how did it get started? How does Bigeye help teams understand and manage their data quality? What is the difference between existing data quality approaches and data observability? What do you see as the tradeoffs for the approach that you are taking at Bigeye? What are the most common data quality issues that you’ve seen and what are some more interesting ones that you wouldn’t expect? Where do you see Bigeye fitting into the data management landscape? What are alternatives to Bigeye? What are some of the most interesting, innovative, or unexpected ways that you have seen Bigeye being used? What are some of the most interesting homegrown approaches that you have seen? What have you found to be the most interesting, unexpected, or challenging lessons that you have learned while building the Bigeye platform and business? What are the biggest trends you’re following in data quality management? When is Bigeye the wrong choice? What do you see in store for the future of Bigeye? Contact Info You can email Egor about anything data LinkedIn Parting Question From your perspective, what is the biggest gap in the tooling or technology for data management today? Closing Announcements Thank you for listening! Don’t forget to check out our other show, Podcast.__init__ to learn about the Python language, its community, and the innovative ways it is being used. Visit the site to subscribe to the show, sign up for the mailing list, and read the show notes. If you’ve learned something or tried out a project from the show then tell us about it! Email [email protected]) with your story. To help other people find the show please leave a review on iTunes and tell your friends and co-workers Join the community in the new Zulip chat workspace at dataengineeringpodcast.com/chat Links Bigeye Uber A/B Testing Hadoop MapReduce Apache Impala One King’s Lane Vertica Mode Tableau Jupyter Notebooks Redshift Snowflake PyTorch Podcast.__init__ Episode Tensorflow DataOps DevOps Data Catalog DBT Podcast Episode SRE Handbook Article About How Uber Applied SRE Principles to Data SLA == Service Level Agreement SLO == Service Level Objective Dagster Podcast Episode Podcast.__init__ Episode Delta Lake Great Expectations Podcast Episode Podcast.__init__ Episode Amundsen Podcast Episode Alation Collibra The intro and outro music is from The Hug by The Freak Fandango Orchestra / CC BY-SA Support Data Engineering Podcast