Streaming Data Pipelines Made SQL With Decodable
Data Engineering Podcast - Un pódcast de Tobias Macey - Domingos
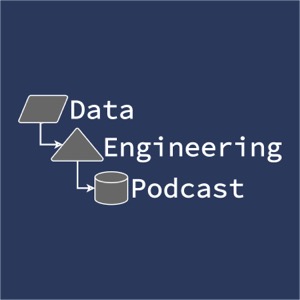
Categorías:
Summary Streaming data systems have been growing more capable and flexible over the past few years. Despite this, it is still challenging to build reliable pipelines for stream processing. In this episode Eric Sammer discusses the shortcomings of the current set of streaming engines and how they force engineers to work at an extremely low level of abstraction. He also explains why he started Decodable to address that limitation and the work that he and his team have done to let data engineers build streaming pipelines entirely in SQL. Announcements Hello and welcome to the Data Engineering Podcast, the show about modern data management When you’re ready to build your next pipeline, or want to test out the projects you hear about on the show, you’ll need somewhere to deploy it, so check out our friends at Linode. With their managed Kubernetes platform it’s now even easier to deploy and scale your workflows, or try out the latest Helm charts from tools like Pulsar and Pachyderm. With simple pricing, fast networking, object storage, and worldwide data centers, you’ve got everything you need to run a bulletproof data platform. Go to dataengineeringpodcast.com/linode today and get a $100 credit to try out a Kubernetes cluster of your own. And don’t forget to thank them for their continued support of this show! Struggling with broken pipelines? Stale dashboards? Missing data? If this resonates with you, you’re not alone. Data engineers struggling with unreliable data need look no further than Monte Carlo, the world’s first end-to-end, fully automated Data Observability Platform! In the same way that application performance monitoring ensures reliable software and keeps application downtime at bay, Monte Carlo solves the costly problem of broken data pipelines. Monte Carlo monitors and alerts for data issues across your data warehouses, data lakes, ETL, and business intelligence, reducing time to detection and resolution from weeks or days to just minutes. Start trusting your data with Monte Carlo today! Visit dataengineeringpodcast.com/impact today to save your spot at IMPACT: The Data Observability Summit a half-day virtual event featuring the first U.S. Chief Data Scientist, founder of the Data Mesh, Creator of Apache Airflow, and more data pioneers spearheading some of the biggest movements in data. The first 50 to RSVP with this link will be entered to win an Oculus Quest 2 — Advanced All-In-One Virtual Reality Headset. RSVP today – you don’t want to miss it! Are you bored with writing scripts to move data into SaaS tools like Salesforce, Marketo, or Facebook Ads? Hightouch is the easiest way to sync data into the platforms that your business teams rely on. The data you’re looking for is already in your data warehouse and BI tools. Connect your warehouse to Hightouch, paste a SQL query, and use their visual mapper to specify how data should appear in your SaaS systems. No more scripts, just SQL. Supercharge your business teams with customer data using Hightouch for Reverse ETL today. Get started for free at dataengineeringpodcast.com/hightouch. Your host is Tobias Macey and today I’m interviewing Eric Sammer about Decodable, a platform for simplifying the work of building real-time data pipelines Interview Introduction How did you get involved in the area of data management? Can you describe what Decodable is and the story behind it? Who are the target users, and how has that focus informed your prioritization of features at launch? What are the complexities that data engineers encounter when building pipelines on streaming systems? What are the distributed systems concepts and design optimizations that are often skipped over or misunderstood by engineers who are using them? (e.g. backpressure, exactly once semantics, isolation levels, etc.) How do those mismatches in understanding and expectation impact the correctness and reliability of the workflows that they are building? Can you describe how you have architected the Decodable platform? What have been the most complex or time consuming engineering challenges that you have dealt with so far? What are the points of integration that you expose for engineers to wire in their existing infrastructure and data systems? What has been your process for designing the interfaces and abstractions that you are exposing to end users? What are some of the leaks in those abstractions that have either started to show or are anticipated? What have you learned about the state of data engineering and the costs and benefits of real-time data while working on Decodable? What are the most interesting, innovative, or unexpected ways that you have seen Decodable used? What are the most interesting, unexpected, or challenging lessons that you have learned while working on Decodable? When is Decodable the wrong choice? What do you have planned for the future of Decodable? Contact Info esammer on GitHub @esammer on Twitter LinkedIn Parting Question From your perspective, what is the biggest gap in the tooling or technology for data management today? Links Decodable Cloudera Kafka Flink Podcast Episode Spark Snowflake Podcast Episode BigQuery RedShift kSQLDB Podcast Episode dbt Podcast Episode Millwheel Paper Dremel Paper Timely Dataflow Materialize Podcast Episode Software Defined Networking Data Mesh Podcast Episode OpenLineage Podcast Episode DataHub Podcast Episode Amundsen Podcast Episode The intro and outro music is from The Hug by The Freak Fandango Orchestra / CC BY-SA Support Data Engineering Podcast