System Observability For The Cloud Native Era With Chronosphere
Data Engineering Podcast - Un pódcast de Tobias Macey - Domingos
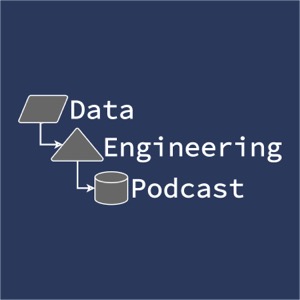
Categorías:
Summary Collecting and processing metrics for monitoring use cases is an interesting data problem. It is eminently possible to generate millions or billions of data points per second, the information needs to be propagated to a central location, processed, and analyzed in timeframes on the order of milliseconds or single-digit seconds, and the consumers of the data need to be able to query the information quickly and flexibly. As the systems that we build continue to grow in scale and complexity the need for reliable and manageable monitoring platforms increases proportionately. In this episode Rob Skillington, CTO of Chronosphere, shares his experiences building metrics systems that provide observability to companies that are operating at extreme scale. He describes how the M3DB storage engine is designed to manage the pressures of a critical system component, the inherent complexities of working with telemetry data, and the motivating factors that are contributing to the growing need for flexibility in querying the collected metrics. This is a fascinating conversation about an area of data management that is often taken for granted. Announcements Hello and welcome to the Data Engineering Podcast, the show about modern data management When you’re ready to build your next pipeline, or want to test out the projects you hear about on the show, you’ll need somewhere to deploy it, so check out our friends at Linode. With their managed Kubernetes platform it’s now even easier to deploy and scale your workflows, or try out the latest Helm charts from tools like Pulsar and Pachyderm. With simple pricing, fast networking, object storage, and worldwide data centers, you’ve got everything you need to run a bulletproof data platform. Go to dataengineeringpodcast.com/linode today and get a $100 credit to try out a Kubernetes cluster of your own. And don’t forget to thank them for their continued support of this show! Modern Data teams are dealing with a lot of complexity in their data pipelines and analytical code. Monitoring data quality, tracing incidents, and testing changes can be daunting and often takes hours to days. Datafold helps Data teams gain visibility and confidence in the quality of their analytical data through data profiling, column-level lineage and intelligent anomaly detection. Datafold also helps automate regression testing of ETL code with its Data Diff feature that instantly shows how a change in ETL or BI code affects the produced data, both on a statistical level and down to individual rows and values. Datafold integrates with all major data warehouses as well as frameworks such as Airflow & dbt and seamlessly plugs into CI workflows. Go to dataengineeringpodcast.com/datafold today to start a 30-day trial of Datafold. Once you sign up and create an alert in Datafold for your company data, they will send you a cool water flask. Today’s episode of Data Engineering Podcast is sponsored by Datadog, the monitoring and analytics platform for cloud-scale infrastructure and applications. Datadog’s machine-learning based alerts, customizable dashboards, and 400+ vendor-backed integrations makes it easy to unify disparate data sources and pivot between correlated metrics and events for faster troubleshooting. By combining metrics, traces, and logs in one place, you can easily improve your application performance. Try Datadog free by starting a your 14-day trial and receive a free t-shirt once you install the agent. Go to dataengineeringpodcast.com/datadog today see how you can unify your monitoring today. Your host is Tobias Macey and today I’m interviewing Rob Skillington about Chronosphere, a scalable, reliable and customizable monitoring-as-a-service purpose built for cloud-native applications. Interview Introduction How did you get involved in the area of data management? Can you start by describing what you are building at Chronosphere and your motivation for turning it into a business? What are the biggest challenges inherent to monitoring use cases? How does the advent of cloud native environments complicate things further? While you were at Uber you helped to create the M3 storage engine. There are a wide array of time series databases available, including many purpose built for metrics use cases. What were the missing pieces that made it necessary to create a new system? How do you handle schema design/data modeling for metrics storage? How do the usage patterns of metrics systems contribute to the complexity of building a storage layer to support them? What are the optimizations that need to be made for the read and write paths in M3? How do you handle high cardinality of metrics and ad-hoc queries to understand system behaviors? What are the scaling factors for M3? Can you describe how you have architected the Chronosphere platform? What are the convenience features built on top of M3 that you are creating at Chronosphere? How do you handle deployment and scaling of your infrastructure given the scale of the businesses that you are working with? Beyond just server infrastructure and application behavior, what are some of the other sources of metrics that you and your users are sending into Chronosphere? How do those alternative metrics sources complicate the work of generating useful insights from the data? In addition to the read and write loads, metrics systems also need to be able to identify patterns, thresholds, and anomalies in the data to alert on it with minimal latency. How do you handle that in the Chronosphere platform? What are some of the most interesting, innovative, or unexpected ways that you have seen Chronosphere/M3 used? What are some of the most interesting, unexpected, or challenging lessons that you have learned while building Chronosphere? When is Chronosphere the wrong choice? What do you have planned for the future of the platform and business? Contact Info LinkedIn @roskilli on Twitter robskillington on GitHub Parting Question From your perspective, what is the biggest gap in the tooling or technology for data management today? Closing Announcements Thank you for listening! Don’t forget to check out our other show, Podcast.__init__ to learn about the Python language, its community, and the innovative ways it is being used. Visit the site to subscribe to the show, sign up for the mailing list, and read the show notes. If you’ve learned something or tried out a project from the show then tell us about it! Email [email protected]) with your story. To help other people find the show please leave a review on iTunes and tell your friends and co-workers Join the community in the new Zulip chat workspace at dataengineeringpodcast.com/chat Links Chronosphere Lidar Cloud Native M3DB OpenTracing Metrics/Telemetry Graphite Podcast.__init__ Episode InfluxDB Clickhouse Podcast Episode Prometheus Inverted Index Druid Cardinality Apache Flink Podcast Episode HDFS Avro Podcast Episode Grafana Tecton Podcast Episode Datadog Podcast Episode Kubernetes Sourcegraph The intro and outro music is from The Hug by The Freak Fandango Orchestra / CC BY-SA Support Data Engineering Podcast