#22 Eliciting Priors and Doing Bayesian Inference at Scale, with Avi Bryant
Learning Bayesian Statistics - Un pódcast de Alexandre Andorra
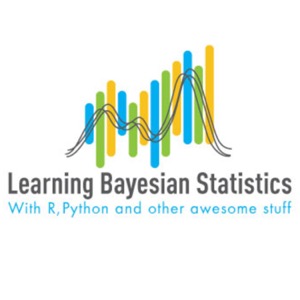
Categorías:
If, like me, you’ve been stuck in a 40 square-meter apartment for two months, you’re going to be pretty jealous of Avi Bryant. Indeed, Avi lives on Galiano Island, Canada, not very far from Vancouver, surrounded by forest, overlooking the Salish Sea.
In this natural and beautiful — although slightly deer-infested — spot, Avi runs The Gradient Retreat Center, a place where writers, makers, and code writers can take a week away from their regular lives and focus on creative work. But it’s not only to envy him that I invited Avi on the show — it’s to talk about Bayesian inference in Scala, prior elicitation, how to deploy Bayesian methods at scale, and how to enable Bayesian inference for engineers.
While working at Stripe, Avi wrote Rainier, a Bayesian inference framework for Scala. Inference is based on variants of the Hamiltonian Monte Carlo sampler, and the implementation is similar to, and targets the same types of models as both Stan and PyMC3. As Avi says, depending on your background, you might think of Rainier as aspiring to be either "Stan, but on the JVM", or "TensorFlow, but for small data".
In this episode, Avi will tell us how Rainier came into life, how it fits into the probabilistic programming landscape, and what its main strengths and weaknesses are.
Our theme music is « Good Bayesian », by Baba Brinkman (feat MC Lars and Mega Ran). Check out his awesome work at https://bababrinkman.com/ !
Links from the show:
- Avi on Twitter: https://twitter.com/avibryant
- Avi on GitHub: https://github.com/avibryant
- Rainier -- Bayesian Inference in Scala: https://rainier.fit/
- The Gradient Retreat: https://gradientretreat.com/
- Facebook's Prophet: https://facebook.github.io/prophet/
- BAyesian Model-Building Interface (Bambi) in Python: https://bambinos.github.io/bambi/
- BRMS -- Bayesian regression models using Stan: https://paul-buerkner.github.io/brms/
- Using Bayesian Decision Making to Optimize Supply Chains -- Thomas Wiecki & Ravin Kumar: https://twiecki.io/blog/2019/01/14/supply_chain/