NLP is not NLU and GPT-3 - Walid Saba
Machine Learning Street Talk (MLST) - Un pódcast de Machine Learning Street Talk (MLST)
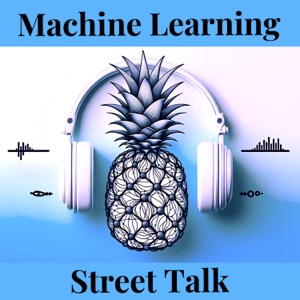
Categorías:
#machinelearning This week Dr. Tim Scarfe, Dr. Keith Duggar and Yannic Kilcher speak with veteran NLU expert Dr. Walid Saba. Walid is an old-school AI expert. He is a polymath, a neuroscientist, psychologist, linguist, philosopher, statistician, and logician. He thinks the missing information problem and lack of a typed ontology is the key issue with NLU, not sample efficiency or generalisation. He is a big critic of the deep learning movement and BERTology. We also cover GPT-3 in some detail in today's session, covering Luciano Floridi's recent article "GPT‑3: Its Nature, Scope, Limits, and Consequences" and a commentary on the incredible power of GPT-3 to perform tasks with just a few examples including the Yann LeCun commentary on Facebook and Hackernews. Time stamps on the YouTube version 0:00:00 Walid intro 00:05:03 Knowledge acquisition bottleneck 00:06:11 Language is ambiguous 00:07:41 Language is not learned 00:08:32 Language is a formal language 00:08:55 Learning from data doesn’t work 00:14:01 Intelligence 00:15:07 Lack of domain knowledge these days 00:16:37 Yannic Kilcher thuglife comment 00:17:57 Deep learning assault 00:20:07 The way we evaluate language models is flawed 00:20:47 Humans do type checking 00:23:02 Ontologic 00:25:48 Comments On GPT3 00:30:54 Yann lecun and reddit 00:33:57 Minds and machines - Luciano 00:35:55 Main show introduction 00:39:02 Walid introduces himself 00:40:20 science advances one funeral at a time 00:44:58 Deep learning obsession syndrome and inception 00:46:14 BERTology / empirical methods are not NLU 00:49:55 Pattern recognition vs domain reasoning, is the knowledge in the data 00:56:04 Natural language understanding is about decoding and not compression, it's not learnable. 01:01:46 Intelligence is about not needing infinite amounts of time 01:04:23 We need an explicit ontological structure to understand anything 01:06:40 Ontological concepts 01:09:38 Word embeddings 01:12:20 There is power in structure 01:15:16 Language models are not trained on pronoun disambiguation and resolving scopes 01:17:33 The information is not in the data 01:19:03 Can we generate these rules on the fly? Rules or data? 01:20:39 The missing data problem is key 01:21:19 Problem with empirical methods and lecunn reference 01:22:45 Comparison with meatspace (brains) 01:28:16 The knowledge graph game, is knowledge constructed or discovered 01:29:41 How small can this ontology of the world be? 01:33:08 Walids taxonomy of understanding 01:38:49 The trend seems to be, less rules is better not the othe way around? 01:40:30 Testing the latest NLP models with entailment 01:42:25 Problems with the way we evaluate NLP 01:44:10 Winograd Schema challenge 01:45:56 All you need to know now is how to build neural networks, lack of rigour in ML research 01:50:47 Is everything learnable 01:53:02 How should we elevate language systems? 01:54:04 10 big problems in language (missing information) 01:55:59 Multiple inheritance is wrong 01:58:19 Language is ambiguous 02:01:14 How big would our world ontology need to be? 02:05:49 How to learn more about NLU 02:09:10 AlphaGo Walid's blog: https://medium.com/@ontologik LinkedIn: https://www.linkedin.com/in/walidsaba/