Biotech startup working with Oracle to innovate for pharma
Research in Action - Un pódcast de Oracle Corporation
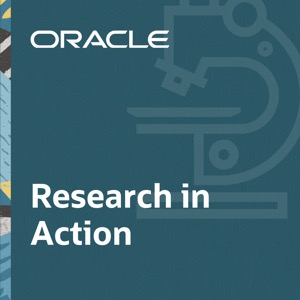
Categorías:
How is academia fostering research that later turns into startup companies? What are new computational powers bringing to in silico drug design? And what is MoveableType methodology and why should pharma be excited about it? We will learn those answers and more in this episode with Lance Westerhoff, President and General Manager of QuantumBio. QuantumBio is a biotech startup operating in the vast field of drug discovery and molecular design. As President and GM, Lance oversees QuantumBio’s day-to-day management including the research, development, and deployment of advanced technology, as well as strategic partnerships and business development. Lance earned his PhD in Chemistry at Penn State University, and he is an entrepreneur, computational biochemist, and published scientist with projects involving the synergistic application of quantum mechanics and molecular mechanics in the life and pharmaceutical sciences. QuantumBio recently earned a Small Business Innovation Research (SBIR) grant from the NIH to run calculations for their MovableType methodology research, which they will be working with Oracle on that research project, and we talk about that and much more in this episode. -------------------------------------------------------- Episode Transcript: 00;00;00;00 - 00;00;26;06 How was academia fostering research that later turns into startup companies? What are new computational powers bringing to in Silico drug design and what is moveable type methods? And why should pharma be excited about it? We'll get those answers and more on research and action in the lead. The leading scene. Hello and welcome to Research and Action, brought to you by Oracle for Research. 00;00;26;06 - 00;01;00;18 I'm Mike Stiles. And today our guest is Lance Wester Hof, who is president and general manager of Quantum Bio. That's a biotech startup that operates in the field of drug discovery and molecular design. Lance oversees day to day management, including the research, development and deployment of advanced technology, as well as strategic partnerships and business development. He earned his Ph.D. in chemistry at Penn State, and he's an entrepreneur, a computational biochemist and published scientist with projects involving the synergistic application of quantum mechanics and molecular mechanics in the life and pharmaceutical sciences. 00;01;00;20 - 00;01;24;09 In fact, Quantum Bio earned a small business innovation research grant from the NIH to run calculations for their movable type methodology. Research. They'll be working with Oracle on that project. So, Lance, we're really glad to have you with us. Certainly. Well, thank you for having me. I look forward to the discussion. Well, listeners, I hope you're ready to get into the weeds because we're going to get into chemistry quantum and all the exciting things that are becoming possible. 00;01;24;12 - 00;01;44;12 And it's all emerging science and technology. So keep listening. You'll be well caught up. But to start, we're always interested in what got you, Lance, and what you're doing. What was that professional and personal journey like? Certainly. Yeah, well, and actually, I when I first started things out or I just started really putting my head around what I wanted to do for a living. 00;01;44;15 - 00;02;06;22 Science was actually pretty far from from the discussion or my thought process I'd actually started is as a semiprofessional professional amateur theater geek, doing a lot of five local theater, that sort of thing. I worked at a local Renaissance fair, you know, those sorts of things that that that people that wanted to go more into the the arts. 00;02;06;22 - 00;02;24;22 If you will, you're really wanted to do. And then one day I was when I was in high school and starting to think about what I wanted to do for a living, it just kind of dawned on me that, you know, you could be the best actor in the world and be very successful as a and have a lot of a lot of great enjoyment. 00;02;24;24 - 00;02;46;20 But if you don't catch a break, you can have all sorts of professional and financial difficulties throughout life. And so I started looking at what classes I did well in in high school or what I was doing well. And at that time I was in 10th grade and of course it was the sciences biology at the time. And at the same time I was I had always been into computers. 00;02;46;23 - 00;03;09;06 And so I think my first computer was a Vic 20, which I believe as I, as I looked up, just came out in 1980. So so that kind of puts it perspective that I was about six years old, and so I knew that I would want to do something with computers, something with biology. So then I started really setting up my my high school career for that, for that sort of background. 00;03;09;06 - 00;03;36;13 I studied some theater on the side. Theater is always fun, but, you know, that was where I focused my energy. Then I went to college. I ended up majoring in biochemistry and computer science with an eye towards doing exactly what I'm doing now. And so my wife always jokes with me that and she knew me then too, that, you know, I wanted to do something that most people, including her at the time, had never heard of before, and that was computational biochemistry or computational chemistry. 00;03;36;18 - 00;03;54;15 And so I spent my years in college, you know, certainly learned a lot in biology. I was I was more focused on the biology versus the chemistry side of things, you know, And of course, like I said, with the comp sci. But then when I went, when I started looking at grad school, I had already met my future advisor at the time. 00;03;54;15 - 00;04;18;14 His name is Kenny Myers began at Penn State at the time and now he's he's moved on as well. But I actually had met him a couple of years before I graduated from college and, you know, started talking to him. And then we ended up I decided that was the lab that I wanted to work in. You know, once I went to Penn State and so as I settled in into graduate school again, that would have been in 1998 when I had started grad school. 00;04;18;16 - 00;04;45;15 By about 2000, 2001, you know, I was really starting to think about and talking to him, of course, at the same time about the possibility of starting a company. And I had already done started some companies back then, back in, I guess you could say the the college years doing, you know, web design for people you know back when the web was very, very young and and just getting started those sorts of jobs. 00;04;45;15 - 00;05;15;02 And so I had already had an understanding of of the basics of of getting a business started. And so at that time, then, you know, Katie and myself and then another person began the companies really to focus on commercializing the linear scaling semi semi empirical quantum mechanics technology from from his lab and spinning that out again as as a company that's really focused on applying these methods to drug discovery working in the pharmaceutical space. 00;05;15;05 - 00;05;42;13 Yeah, I've been calling quantum bio a startup, but it's actually pretty established. It's spun out of Penn State in 2002. How did the company come to be and what does it aim to do? What were your highest aspirations for it? Well, I'll tell you, when you're around that long and you've done, you know, a lot of say, ups and downs, we we we always joke with our investors and everything else on the topic that you're really you know, there's a lot of trial by fire when it comes to entrepreneurship and that is part of the process. 00;05;42;13 - 00;06;07;00 And so you become very comfort, comfortable with trying different things, seeing what works, what doesn't work, and learning from mistakes and moving forward. And so when we first spun out the company, it was very focused on a we have a patent that's associated with it, which was a quantum scoring based methodology that again was published probably about that same time frame. 00;06;07;00 - 00;06;28;09 You know, you know, early 2000s. We thought this was going to be the greatest technology that was going to be known to man or whatever and was going to be very successful in pharma. And I think what we learned was that, you know, trying to just develop a academic software package and commercialize it, it's well, it takes a lot more than just a good idea. 00;06;28;10 - 00;06;50;22 You know, you really need to understand, you know, how software is put together. You need to not necessarily focus on it from an academic perspective, answering academic questions, if you will, and really focus more on your client is what the client really needs to do and how much time and effort they're willing to spend on that. And so that's how we learned. 00;06;50;22 - 00;07;10;11 We had a couple of hard lessons along the way, you know, that, you know, these things had to evolve a little bit more, so on and so forth. And so, you know, we certainly but the good news was at the same time we were bringing on clients and, you know, we've we've had made a lot of friends, you know, a lot of folks that we could work with, collaborators, so on and so forth. 00;07;10;13 - 00;07;41;25 And then over the years as we really put our heads around this and understand how things had to progress, I begun to work with the the National Institutes of Health, and I took over the general management of the company at that time and then really focused on raising funding specifically for development of new technologies. And so we've been able to raise probably on the order of about 8 million or so dollars from the Spire program over the over the last several years. 00;07;41;27 - 00;08;12;12 And again, that is focused very specifically on development of new technologies for pharmaceutical research. And we also then at the same time, we expanded beyond just the scoring methodology that we had done, and now we're in the free energy space, the X-ray crystallography space, the nuclear magnetic resonance space. So we've been able to, you could say, grow that that nugget or completely redevelop that nugget and then now expand to into a different lot of different applications. 00;08;12;20 - 00;08;36;05 And that then becomes our key. Then for from a business perspective is now we're focused very much on applications of these technologies. And in order to solve problems, you know, the more interviews we do on the show, the more I'm seeing startups come out of academia as some kind of deliberate ecosystem that's been set up where colleges are basically incubating research startups that then go on to take off. 00;08;36;05 - 00;09;00;08 What what are the universities get out of that? Well, I mean, I think it obviously depends on on a case by case. You know, there's but but in terms of the generalities, those sorts of deals can take a lot of different looks, I guess you could say. So in our case, you know, most of the focus was that you would be bringing out a technology that was developed in the lab. 00;09;00;10 - 00;09;23;08 Again, as I already mentioned, an academic way to solve a problem is oftentimes different than a industrial way to solve a problem. So there's a lot of that R&D that needs to go into developing a technology. And so that's that's one aspect. Certainly the and so therefore, getting that technology out to more people, you know, tends to be a key benefit for universities. 00;09;23;11 - 00;09;48;14 I think the other, of course, you know, is it comes down to a monetary, you know, very oftentimes thanks to the by double act and so on and so forth, there is a requirement that they take this technology and put it out to the world and actually take some sort of monetary value. And so you get a certain amount of of ownership in that in whatever downstream company comes about. 00;09;48;16 - 00;10;18;25 And so that's how generally universities, you know, develop, they pay for their intellectual property offices and so on and so forth by actually investing in those companies. And usually not necessarily, again, invest in cash as much as investing technology into those companies. And then they get they get a certain percentage of the ownership. And so as we as we move forward, then the the certainly it's oftentimes students, grad students, postdocs, faculty, they get the experience and enjoyment of spinning out of business. 00;10;18;25 - 00;10;41;11 So therefore they get some of that training that goes along with it. But then I think also the university gets some some financial benefit then as well. Well, how do you think that relationship is working? Because entrepreneurs are very unique creatures who put it that way. And a lot of PhDs aren't really entrepreneur material. Or better to say, it's not something that comes naturally to them or that they aspire to be. 00;10;41;13 - 00;11;04;08 That's got to be hard. Throwing themselves into business, especially in something like pharma, which is really regulated and competitive. Right, Right. Well, it actually it's interesting. I would actually argue that actually very oftentimes PhDs, especially in the sciences, I again, I can't really speak for, you know, the humanities, but in the sciences very often they are actually entrepreneurial in terms of their spirit. 00;11;04;08 - 00;11;26;04 Now, again, that might manifest itself in different ways. So a great example of that is a professor at a research university. They generally need to raise their own funding to support their labs. They are extremely it's really important that they go out and do quote unquote marketing in the form of going to conferences and conventions and and writing papers and so on and so forth. 00;11;26;06 - 00;11;50;15 And so that that entrepreneurial spirit, I think, is actually pretty strong. And certainly anybody that's in the sciences and, you know, using the scientific method is, you know, already pretty comfortable with risk and uncertainty because, of course, a lot of what we do stems from hypotheses that some of them don't work out. And so, again, that's something that, you know, a lot of entrepreneurs, you know, would be would understand very well. 00;11;50;17 - 00;12;15;02 And so I think that there is a lot of that spirit. I think the difference then oftentimes is, you know, a lot of folks maybe don't necessarily understand a priori or at the beginning of, well, how do I actually raise funding, raise my own capital in order to develop a new a new company out of that? And so it's those aspects that I think where the university can be a really big benefit. 00;12;15;04 - 00;12;38;27 You know, they they kind of provide that little that little test bed or that little seed bed, if you will. They allow that the people to take risks, that it's a little harder to do if, again, you're out on your own. And so I think that that ends up being where a lot of the benefit comes from. And I think that therefore, then they can really kind of play to those strengths and then grow from there and build out from there. 00;12;39;00 - 00;13;04;29 In terms of the pharmaceutical space, absolutely, very highly regulated. That tends to be a an impediment often. I mean, I think it certainly has benefits, but I think the the, the minus of it is you generally have to have a lot more infrastructure that can support understanding of those regulations, understanding of how those those regulations work, how that game is played, quote unquote. 00;13;05;01 - 00;13;24;28 And so I think that part does tend to be a little more difficult. And again, that is where, you know, a university or even just a corporate partner can take a lot of that push, a lot of that heft in terms of competition. You know, I think that's something that I think we we all like and appreciate. These things should be highly competitive. 00;13;25;00 - 00;13;48;26 But the regulation is something that, you know, like I said, there's always this expense that goes along with it and legal fees and so on and so forth. Computational drug design or in silico has shown promise in enhancing the success rates of drug candidates and saving time and money. Now, of course, it can't replace experimental bench work, but in silico platforms like Quantum Bio, they're starting to be seen even by regulators as having a lot of potential. 00;13;48;28 - 00;14;13;21 I mean, the NIH is even funding you. What is so exciting about in Silico drug discovery and design and and why are folks excited about quantum bio? That's a really you know, I think it goes to a core question or a core core benefit that you're absolutely right. I mean, in Silico back, as I mentioned at the beginning, that back in the day was really not something that people fully understood. 00;14;13;28 - 00;14;39;17 They saw maybe a lot of promise there, but there was a lot of concern, you know, is the are the computational methods just going to replace all all lab bench work? And of course, that's nonsense. Of course, lab bench work is is is critical as well. And at the same time, there's a lot of evolution still going on in the field where we were still trying to fully understand how to. 00;14;39;17 - 00;15;11;12 And I think we're still fully trying to fully understand how proteins and ligands interact with each other, how all these different molecules interact with each other. And by expanding that or as that continue to evolve, if you will, and mature, then it really has now become a core aspect of the of any pretty much any pharmaceutical company out there is likely going to have, you know, a number of computational chemists that are working on projects on a day to day basis. 00;15;11;15 - 00;15;32;16 You know, this is now a critical part, a core part of the drug discovery process where again, back back before it was probably more something that folks kept an eye on. They they wanted to see what sort of ideas would be coming out of it. But oftentimes is that as the saying would go, you know, you're you're only going to give them a certain amount of time to give a result. 00;15;32;16 - 00;15;59;02 And if if not, I'm just going to go ahead and go to the lab and make it anyway. And so methods like the ones that we work with and and develop have now, really, like I said, they've become ubiquitous. You know, they are something that's that's part of the drug discovery or pharmaceutical space. And now then where we focus them is laser on very specific specific solutions to very specific types of problems. 00;15;59;04 - 00;16;19;03 And so and so therefore, we play to our strengths and then we very oftentimes then partner with other software companies that then are applying to theirs. And so in in our world, collaboration between pharma, between other software companies is is just part of the day to day. You know, we need to make sure that our software works well with theirs. 00;16;19;03 - 00;16;43;08 They're doing what they do really, really well, and we're doing what we do really, really well. Then that then provides a solution or a set of solutions that really gets added into a toolbox for the pharmaceutical space. And so a firm before and so a practitioner in the pharmaceutical world will have any number of tools. Ours is one of them that that would be solving the types of problems that they need to solve on a day to day basis. 00;16;43;11 - 00;17;10;10 Pharma, it seems to me, moves really slowly and cautiously, sometimes frustratingly slow toward emerging innovations. And silica has been around a long time, so they got used to that. But now computational power has made it a real for something with enormous potential. So how is pharma acting now? Are they do they get it? Are they fully adopting it to modernize and speed drug discovery? 00;17;10;12 - 00;17;34;01 Yes. Oh yeah. I think clearly that's the case. You know, I think now it's it's really like I said a few minutes ago, I mean, I think it's it's it's a critical part of the R&D process now. I would be surprised if there's any pharmaceutical company doing direct or R&D efforts in the world that that doesn't have, you know, at least some computational chemistry muscle. 00;17;34;08 - 00;18;05;08 Because, again, I think what what a lot of it is we can test we can test hypotheses in the computer that are either difficult to test in at the lab lab bench or that are just expensive or that are, you know, maybe a little, little early in the sense that things that we might we try to what we want to try to build a hypothesis as far as possible before you start throwing a whole bunch of chemicals at it, you know, chemicals and the people and so on and so forth. 00;18;05;08 - 00;18;42;20 Obviously there are costs. And so what you try to do is you want to optimize their time as much as possible, not maybe flush quite as many chemicals down the drain and really focus on solving the cases or addressing the the solutions that you really need to address in order to understand how a molecule is interacting. And so if you think about it this way, a, the drug discovery process takes, you know, billions of dollars and they go through untold hundreds, thousands of potential compounds to finally get to that one compound that's going to be the winner. 00;18;42;22 - 00;19;12;09 Anything that we can do or to to understand and better impact decisions, the better for the whole process it gets. Obviously, the process hopefully gets cheaper, but much more importantly is it gets more, more efficient. We start solving problems quicker so that way we can get that drug to market much, much quicker. Do The pandemic changed the dynamic in terms of how important it is to get to discovery, get drugs to market really, really quickly? 00;19;12;09 - 00;19;47;05 I mean, was that was it any kind of wake up call for speeding discovery? Absolutely. Yeah. I think the pandemic was a you know, it really showed, I think, where computational chemistry can can, you know, really adds to the whole whole process of drug discovery. And so, I mean, Grady, just on the very simplest level, even where as we had as we were doing was shut down in the very early stages of the of the pandemic, you know, people a lot of pharmaceutical companies had to also shut down. 00;19;47;05 - 00;20;10;20 They had to you know, people couldn't come in to the lab. They couldn't work in the lab. So even on the simplest level, computational chemists could continue working. You know, we didn't have to go into the lab. You know, we continued to do the the modeling, the the R&D to really understand what was going on with COVID and understand how the virus is working and getting structure very, very early on. 00;20;10;20 - 00;20;46;22 And so I can remember very early on being a party to online virtual meetings, conferences, meetings with clients and partners and collaborators where we were actively discussing how are we going to solve this problem, How can we as a field add value and solve this problem while, again, everybody else was effectively locked down in their houses and so very quickly there was structure that had come out of this this these these sorts of efforts where we understood what the structure looked like very early on. 00;20;46;24 - 00;21;15;13 And that was just an amazing there's a whole websites and papers and books that are that are dedicated to that topic of just the process of coming up with structure, understanding how this thing was going to work, and then therefore then we could take that and move forward into here are the therapeutics. At that time we didn't necessarily fully recognize that we would get to any sort of vaccine as quickly as we did, and I think that was an amazing accomplishment in itself. 00;21;15;15 - 00;21;34;02 But we were at that time we were still talking about, well, this is the way that this virus works. How can we develop therapeutics in order to address it? And so that that effort, you know, I think was a definite success. And it really showed what computational chemistry can do, especially, like I said, in a very hard situation that we were dealing with as a world. 00;21;34;05 - 00;21;59;28 You're now each research grant is for your movable type methodology research. Now, I don't know what that is, but I know it's patented so I can't steal it yet. So tell me what that methodology is. Certainly. Certainly, Yeah. So this this methodology was actually one of the kind of we were alluding to earlier was it was developed in the lab, in the academic lab originally, and we licensed it from Michigan State University. 00;22;00;03 - 00;22;23;14 At that time. We took it, you know, licensed it, redeveloped it, re-implemented it from the ground up, so on and so forth, actually partnered with the university and to really get their expertise as we were doing it. So I think that was a win and that was certainly a showed a nice core collaboration between a university and an industrial partner and how that can be very successful. 00;22;23;21 - 00;22;44;21 And from there then we've we've now taken that technology and brought that to the world. And now it is something that we're marketing directly to pharma, to biotech, to CEOs, consultants, that sort of thing. Now the question to answer the question of what it is or what it's doing, it's effectively it's it's what's called a free energy simulation method. 00;22;44;23 - 00;23;06;09 The way that that that modeling generally works are simulations. Work is they usually take a lot of CPU time, a lot of GPU time actually, and you model how to molecules interact with each other over time. And when I say over time, it's you're generally modeling nanoseconds or picoseconds worth of time that's in the solution, if you will. 00;23;06;16 - 00;23;40;12 But this is taking weeks or excuse me, I should say weeks anymore, but, but certainly hours. The days in order to get to get to those results, it's something that then you're modeling those interactions and how that that these two molecules or three or four or however many are interacting through time. And what that gives us an understanding of is when it's happening this way and in the quote unquote test tube, in this case a computational test, you or the in silico test tube, it mimics what is likely happening also within the body. 00;23;40;14 - 00;24;19;23 And so by doing that, we can inject the different and I say again, a kind of error code, inject this all obviously computationally or virtually inject a different molecule into this solution and allow that interactions, those interactions to happen. And by modeling those those changes over time, we can see that this interaction with or this particular molecule and again, I'm just talking about the protein ligand space just for this example, but a little molecule with a big molecule, how those two are interacting with each other and if they interact and actually bind together, well then that's, that's a possible drug. 00;24;19;26 - 00;24;35;17 Yeah, we might we might have just found $1,000,000,000 drug alternatively, and this is what usually happens is it falls apart. You know, it didn't, it didn't actually interact. And so that those are the failures, those are the things that then you would tell the bench chemist, I probably don't make that one, make this one, this one looked like it interacted. 00;24;35;17 - 00;24;54;05 Well, that one didn't. But the problem is, as I alluded to, that takes a lot of time that that interaction, all of those interactions takes a lot of time, a computational time. And of course, time is is expense both in terms of the the lab chemist that's maybe waiting for an answer and that person's only going to wait so long. 00;24;54;08 - 00;25;22;01 Also, on the other hand, and it's just the amount of, you know, paying the keep the lights on there, the air conditioning, so on and so forth that goes along with the with the computer. And so what we do then what moveable type does is it says, well, now hold on a minute, Do we need to do all of that modeling or can we start with some key starting points and that maybe maybe they would be what are what we call doc positions or just positions of that ligand within the active site? 00;25;22;03 - 00;25;48;20 Or maybe we actually run shorter simulations and we take snapshots and then we take those points and we say, okay, now we're going to use our method again, what's called movable type to basically smear or blur the interactions between the protein and the ligand In this case. What that does then is that effectively mimics that binding. It mimics all of these local very, very local sampling interactions. 00;25;48;20 - 00;26;22;05 And so what that does for us is we don't have to do the long simulations in order to get an understanding of binding between that that protein and ligand. And therefore our hope would be awe. And what we're seeing is that we can now inject that that that virtual drug in quicker get a quicker understanding of is this good or bad, is this a good molecule or a bad molecule from a binding perspective and then pass that that information down, then to the the medicinal chemistry of the bench chemist to then then go ahead and make the winners versus the losers. 00;26;22;12 - 00;26;55;11 And so the methodology itself is something that then can answer these questions much quicker, that then we can then move a potential drug down that line much, much faster. As I mentioned, Oracle is partnering with Quantum Bio on this NIH research. But in in what way? What does that look like? So that so that's that's I think a core critical aspect that I think is, you know, hopefully, you know, beginning to come out of this this discussion is that a lot of what we do and a lot of what our our field does is a lot of collaboration. 00;26;55;16 - 00;27;24;12 It's a lot of going back and forth between between different entities. No one person or no one organization tends to bring everything to the table and so in our case, what we need is we need the computational muscle. You know, we have the the the algorithms, the software, if you will, of the part of this aspect. But what all of our our methods need is, is some level of modeling or simulation. 00;27;24;15 - 00;27;56;27 And that does take a certain amount of CPU time. Now, we could of course, go and buy a bunch of computers and we of course have some computers that, you know, cluster of our own that we, that we can do some basic testing on. But in this particular project where we're running not just, you know, ten or 20 dynamics calculations in order to get those little snapshots, but we're actually running hundreds of dynamics calculations where now again, each one because now as part of the project, we're comparing to conventional dynamics calculations. 00;27;56;27 - 00;28;23;06 So we still have to run those long simulations to give us a baseline. But then what we do then is we take a take snapshots along the way, with the idea being that hopefully, depending upon the success of this project, we won't need to run all of those long calculations. We could run maybe 20% of the time and still get similar predictive capabilities versus, you know, what the conventional method is. 00;28;23;12 - 00;28;53;00 And so what Oracle is bringing to the table is that ability to run these that computational muscle, that hardware, and that goes along with it, of actually running all of those calculations in parallel. Okay. So now I'm going to put us in our nonexistent time machine and fly forward to the day all of your research is done. If you prove that 90% of the time quantum bio's methodology can get the same or better results with just 25% of the computational time, what does that mean? 00;28;53;07 - 00;29;15;04 What would a Fama exact see? And that that gets them really, really stoked. So that that is, that is savings in terms in a number of different ways. One of course is is the obvious one and that is the just the cost of running computers or cloud computing or again, building their own computers and having to call them and show them and so on and so forth. 00;29;15;11 - 00;29;35;15 So that's the obvious one. That's the obvious best benefit. But there's also an opportunity cost that as I've as I've alluded to, is that if you can't answer a question, if you can't answer a medicinal chemist's question fairly quickly, they will go ahead and make the compound anyway. Of course. I mean, they still have to do their job. 00;29;35;15 - 00;29;56;07 They still have their own hypotheses that they need to test. And so we want to really address both those costs that if a if a calculation is going to take a conventional again, not with movable type, but a conventional calculation may take, you know, again, hours to days, you know, maybe a week or so potentially to get to get an understanding. 00;29;56;09 - 00;30;21;21 That's something that a lot of folks it becomes just problematic to wait for. You know, why not? Why don't we just go ahead and move forward? Why should we wait that long to test hypothesis so we can cut that time down to basically over almost over lunchtime, you know, over a short period of time. Now you're now you've actually given the tool, a tool that then the client can now solve more hypotheses. 00;30;21;27 - 00;30;52;29 They can try more hypotheses, they can they can squirt more virtual chemical into a virtual box and get their answer quicker to then separate the the garbage from from the good stuff during that that downstream process. And so that's that's really where we're coming in is and that's where there's a huge amount of upside benefit to that pharmaceutical company by cutting down that cost, not just the cost but the time that it takes to get to that. 00;30;53;04 - 00;31;15;26 That final answer when researchers are thinking about, okay, what tools am I going to need to use? Am I stuck with a legacy system? Should I use on premises or go with the cloud? Will a hybrid strategy work? What were your answers to those questions? What did you see as the pros and cons of those choices? Well, so I think I mean, I think the answer is probably in this in the short term is all of the above. 00;31;15;28 - 00;31;41;16 You know, I don't think anybody's looking for necessarily a panacea that that this is the one thing that's going to work for everyone. What I do see is that when it comes to having on site clusters, like I said, they do serve a purpose. We we have one of our own as well. That they are a benefit when it comes to that very quick turnaround testing, you know, almost like that workflow testing, making sure that things are working the way that that they're supposed to work. 00;31;41;19 - 00;32;08;28 But when things shift to production, it doesn't matter where that that hardware resides really as long as the the machine is secure and the the partner is secure, which again, Oracle would be, would be, you know, a partner that would provide that security where we can submit those jobs from anywhere as we were talking about back in the with the COVID discussion, people would be literally sitting on the beach and they would be running calculations. 00;32;08;28 - 00;32;29;22 You know, it really doesn't matter to a computational chemist where that hardware is. We have long since done away with the idea of of that. It has to be a local workstation or a local machine and so I think to computational chemists I think it yeah, the cloud definitely makes a lot of sense and, and that's certainly where I think we see a lot of benefit. 00;32;29;25 - 00;32;52;02 The other side of it then too of, of having on the cloud is then generally someone else is taking care of maintaining the system, upgrading the system both on a hardware and a software basis, addressing again, security needs, all of those that infrastructure and that overhead definitely tends to go out the window and we worry about or we we addressed that the partner is taking care of that. 00;32;52;04 - 00;33;11;08 When it comes to the I guess you could say the the management or what what magic management tends to see then is they might see things a little differently where they would they might see it as well. Yes, that's all a benefit. But then they, they still sometimes are in the the belief of risk, you know, of risk management. 00;33;11;08 - 00;33;43;25 Is the cloud more risky than just having it on a local and a local cluster? And that, I think, is probably a good debate. It's debatable. I do. I would say that one benefit of, you know, getting a cloud provider, somebody an organization like Oracle or some of the others that are focused almost exclusively on make it, making sure that they're maintaining a good secure system, you know probably might be better than your, you know, the the local i.t person that you may have hired for you to take care of your own, your own machine. 00;33;43;25 - 00;34;12;03 So, you know, so so my point is that, you know, I think that there's a lot of benefits to, to the cloud. I think that's certainly where things are going. There's, you know, maybe a little bit of concern and again in the in the farmers space about risk. But I think that is really starting to fall away as as more and more of of us vendors, myself included, are shifting a lot of our attention on to the cloud and allowing clients to run things on the cloud. 00;34;12;03 - 00;34;34;04 I think it's it's like anything else, it's it's a feedback loop. A company's success running on the cloud. They're going to continue to run it on the cloud, and especially as they can push through more and more hypotheses that much quicker. So just like computational power took in Silico to a whole other level, now we have this new great leap forward or backwards or sideways, depending on what you think. 00;34;34;04 - 00;34;56;21 With AI, what is under hyped and overhyped about emerging technologies like AI and quantum computing, for that matter? Yes. So, you know, these are these are certainly two very, very hot topics. You know, I think that in some respects, especially when it comes to quantum computing, I think the that one is still, I think a technology of a of a whole lot of promise. 00;34;56;21 - 00;35;16;07 And we're not sure necessarily where it is all going to go. I mean, there are there are obvious benefits of the ideas of, you know, being able to solve multiple hypotheses at once and do things in a Uber parallel level when it comes to quantum computing. So I think that is it is going to happen, but the progression is is on the slower side. 00;35;16;07 - 00;35;48;08 I mean, we were we were talking about quantum computing back in probably discussions we're having is happening in the lab in 2000. You know, so that's something we've certainly been talking about for a long time. The question has always been, you know, where it's going to be. And I think it's a it is a slower moving evolution and we're going to see where things are in the next 510, you know, so so on in terms of artificial intelligence, that's that's here And now, you know, most folks in in certainly in my space is become a tool in the toolbox. 00;35;48;10 - 00;36;09;00 You know, I think everybody sees the strengths of it. Everybody sees the the abilities of it. They see it also see the weaknesses of it. You know, we're still falling for the interpolation versus extrapolation issue that, you know, AI is pretty good at at solving problems. It's already seen it's it's a little harder to use it to solve solve problems it hasn't seen. 00;36;09;02 - 00;36;30;19 But that being said, it is now something that people use to push their their hypotheses through a little bit quicker or get a better understanding of what they should be studying. And that would be the go to kind of saying or belief is A.I. isn't going to necessarily take your job. What it is probably somebody that uses A.I. is going to take your job. 00;36;30;21 - 00;36;56;10 So we've talked a lot about computing power. And you know, as we've seen, our incredible computing power is now in just about everybody's hands. And in research, we're starting to hear more about patient led research or citizen science. So I guess my question is, what place do you think citizen science has in research? Is it is it real or is it mostly just pretending to let non-scientists have a role? 00;36;56;12 - 00;37;19;25 No, I think it I mean, I think it's real. You know, I think they're it's like anything else. There are pluses and minuses. I think there's it's always a plus to, You know, for folks, this is an, if you will, the person on the street, the layman or whatever term you want to use to better understand the scientific method and the process and what we're really doing, what scientists are really doing. 00;37;19;25 - 00;37;51;20 And very often it's kind of like developing a program or software. The best way to learn a programing language is actually to sit down and do it, you know, from a just a day to day experience perspective. I think the more people understand what science is, what it's doing, the process, that's what's behind it, the better, I think where the, you know, some of the really neat things that citizen scientists or citizens bring or the the layman brings, if you will, they bring a different perspective. 00;37;51;21 - 00;38;17;23 You know, and I think that perspective is a benefit. Things that we have to be a little more careful about, I think, is that your folks can be wrong, scientists can be wrong. Anybody can be wrong when we're going through and part of the scientific method is actually an in embracing the potential of being wrong. You're actually saying that, yes, this is something that we could be incorrect on when we're trained for that. 00;38;17;25 - 00;38;33;29 You can find some difficulty, I guess if you're if you're a, you know, someone that really starts getting behind what they believe, sometimes it can be difficult to shake them. That could be a maybe what I'm trying to say is maybe an embrace of your bias. There's a certain amount of bias that that we work into. Anything is the scientists. 00;38;33;29 - 00;38;57;12 I think they they could end up falling into that bias maybe a little bit stronger. But what do you find yourself thinking about most these days? What's an either looming question in your mind or what's something that maybe you wish researchers like our audience knew? Oh, yeah. I mean, I think one certainly right now just with how, you know, I don't want to go into unnecessary politics and that sort of that sort of aspect. 00;38;57;12 - 00;39;19;08 But I think, you know, something I wish that, you know, a lot of people did understand was that, yes, scientists are people like anyone else, but we don't necessarily know all the answers. It's not about knowing all of the answers. What it is is about using a system, using a method in order to figure out those answers, something that I certainly think about a lot. 00;39;19;10 - 00;39;38;00 You know, and it kind of what we were just talking about a few minutes ago is that how to get more people to understand that method and how that method works and really address the types of questions that the big questions that we have as a society. You know, these these this method is the way to do it. 00;39;38;06 - 00;40;02;00 And, you know, I think we certainly have a great track record in solving problems. Do you think that faith in science and particularly in public health officials took a hit during the pandemic? I mean, people put a lot of stock in what they say. And if they are coming out there and confidently getting it wrong, what kind of damage does that? 00;40;02;03 - 00;40;23;06 I think that if if folks who understood what again, the process of science is all about, I don't think that that it would have been nearly as hard. But yes, I would say that there there has been a hit and I don't think that's necessarily all that controversial at this point. You know, and I think that is something that does need to be addressed. 00;40;23;08 - 00;40;46;06 There has to be a recognition that, for one, mistakes were made. I think there's also a recognition that part of that is in better communication. You know, people are smart enough to understand risk. They're smart enough to understand what is and is not risky to them. And so I think that was probably the part that that really was was undermined the most. 00;40;46;13 - 00;41;12;10 A great analogy that we use a lot, actually, when we're talking about predicting molecules and predicting binding is there used to be a time when when a computational chemist was expected to come in and say, this is the right answer and this is the wrong answer, and that is really started to evolve now to actually I would say that that just started it's now has become more looking almost like the weather report analogy to the weather report. 00;41;12;10 - 00;41;35;27 Weather report talks. Yeah. You don't you don't usually watch Al Roker or whoever you're going to watch in the morning and they tell you that it's going to rain. What it's usually is, is some percentage. It's a percent chance. And so I think people do have an understanding of risk or they can understand risk, but the health officials need to appreciate that and they need to be honest with what the risks are. 00;41;36;00 - 00;41;53;12 Maybe focus more on that perspective, allow people then to to make choices that are going to work for them. Well, that's thanks again for being with us today. I'm really glad you chose science over acting, because if you dare, you'd be walking a picket line right now and you'd probably be very hungry. That's right. It might be. That might be the case. 00;41;53;16 - 00;42;13;20 Well, I'm sure we're going to bring you back because we do want updates on that research project you're doing with Oracle. If want to learn more about you or quantum bio, how can they do that? Absolutely. We are certainly we're we're on LinkedIn as as most folks in our field are. So that's always a good place. Certainly if you Google Quantum bio, you'll you'll come across our our website. 00;42;13;23 - 00;44;33;25 Most of all of our updates are there and certainly you're welcome to subscribe to our list through that. Then we get announcements, then that sort of thing of, of what we're doing at the time, from time to time. All right, perfect. If you are interested in how Oracle can simplify and accelerate your research, check out Oracle dot com slash research and make it a point to check out the next research in action.