Accelerating Drug Discovery Using Machine Learning With TorchDrug
The Python Podcast.__init__ - Un pódcast de Tobias Macey
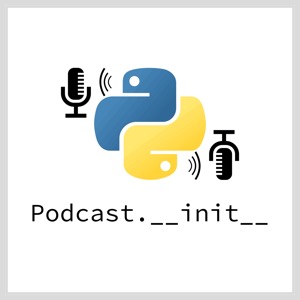
Categorías:
Summary Finding new and effective treatments for disease is a complex and time consuming endeavor, requiring a high degree of domain knowledge and specialized equipment. Combining his expertise in machine learning and graph algorithms with is interest in drug discovery Jian Tang created the TorchDrug project to help reduce the amount of time needed to find new candidate molecules for testing. In this episode he explains how the project is being used by machine learning researchers and biochemists to collaborate on finding effective treatments for real-world diseases. Announcements Hello and welcome to Podcast.__init__, the podcast about Python’s role in data and science. When you’re ready to launch your next app or want to try a project you hear about on the show, you’ll need somewhere to deploy it, so take a look at our friends over at Linode. With the launch of their managed Kubernetes platform it’s easy to get started with the next generation of deployment and scaling, powered by the battle tested Linode platform, including simple pricing, node balancers, 40Gbit networking, dedicated CPU and GPU instances, and worldwide data centers. Go to pythonpodcast.com/linode and get a $100 credit to try out a Kubernetes cluster of your own. And don’t forget to thank them for their continued support of this show! Your host as usual is Tobias Macey and today I’m interviewing Jian Tang about TorchDrug Interview Introductions How did you get introduced to Python? Can you describe what TorchDrug is and the story behind it? What are the goals of the TorchDrug project? Who are the target users of the project? What are the main ways that it is being used? What are the challenges faced by biologists and chemists working on development and discovery of pharmaceuticals? What are some of the other tools/techniques that they would use (in isolation or combination with TorchDrug)? Can you describe how TorchDrug is implemented? How have you approached the design of the project and its APIs to make it accessible to engineers that don’t possess domain expertise in drug discovery research? How do graph structures help when modeling and experimenting with chemical structures for drug discovery? What are the formats and sources of data that you are working with? What are some of the complexities/challenges that you have had to deal with to integrate with up or downstream systems to fit into the overall research process? Can you talk through the workflow of using TorchDrug to build and validate a model? What is involved in determining and codifying a goal state for the model to optimize for? What are the biggest open questions in the area of drug discovery and research? How is TorchDrug being used to assist in the exploration of those problems? What are the most interesting, unexpected, or challenging lessons that you have learned while working on TorchDrug? When is TorchDrug the wrong choice? What do you have planned for the future of TorchDrug? Keep In Touch tangjianpku on GitHub @tangjianpku on Twitter Website LinkedIn Picks Tobias Rope refactoring library Jian Attending conferences once the pandemic is over Closing Announcements Thank you for listening! Don’t forget to check out our other show, the Data Engineering Podcast for the latest on modern data management. Visit the site to subscribe to the show, sign up for the mailing list, and read the show notes. If you’ve learned something or tried out a project from the show then tell us about it! Email [email protected]) with your story. To help other people find the show please leave a review on iTunes and tell your friends and co-workers Join the community in the new Zulip chat workspace at pythonpodcast.com/chat Links TorchDrug Mila Yoshua Bengio Alphafold Few-shot learning Metalearning PyTorch Geometric DeepGraph Library NetworKit Podcast Episode graph-tool Podcast Episode The intro and outro music is from Requiem for a Fish The Freak Fandango Orchestra / CC BY-SA